Databricks for Advanced Analytics
Unlock content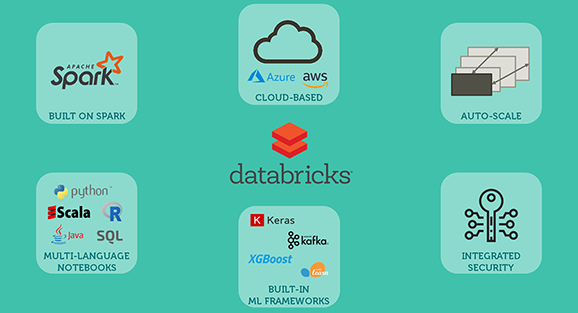
In an ideal world, data scientists would always be handed perfectly clean data, all of it aggregated to exactly the right level, and with all of the features laid out exactly as needed for modeling. Unfortunately, that’s not how the world works. Data is messy. Often times, we don’t know exactly what we need until we start building the model, which requires us to cleanse and transform the data as we tune the model.
Enter Databricks. The first time I encountered the software, during a Microsoft partner training event, it was introduced to me as a statistics and machine learning platform. But not long afterward, while working on a project that involved loading Salesforce sales pipeline data for Power BI reporting, I had the opportunity to use Databricks as a data transformation tool. This multipurpose nature is the reason for the software’s strong appeal, as it offers programmers an expansive toolkit that caters to their specific needs and preferences.
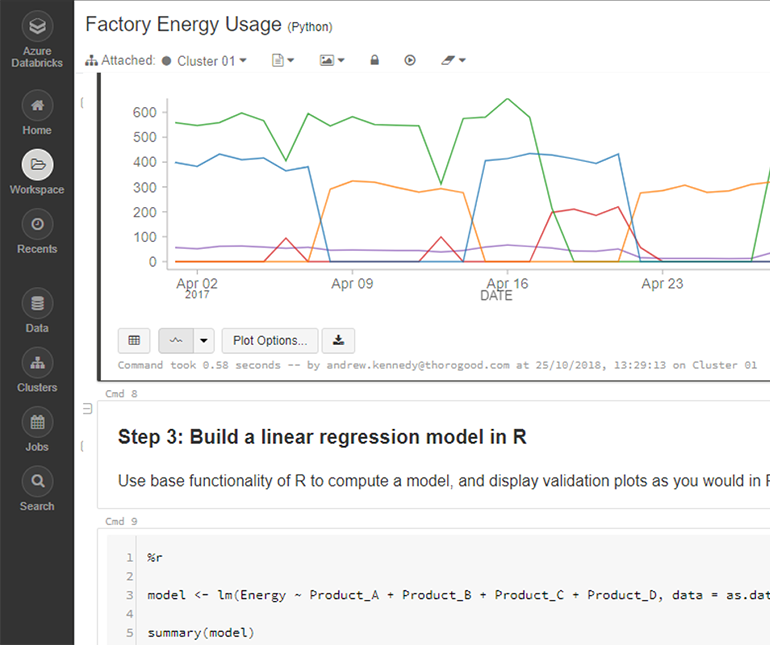
Not only does Databricks enable users to utilize SQL for simple filtering or Python for more complex transformations, it also offers R as an option, which happens to be my default choice for statistical modeling, for the simple fact that it’s what I learned at university. Furthermore, Databricks combines multiple scripting languages in a Notebook format, with simple point-and-click chart options that display data in a way that allows a user to quickly view trends and patterns. This makes a lot of sense for advanced analytics analysis.
If you want to see Databricks in action, check out the demos I put in our recent webcast, or drop me an email if you’d like to discuss how it might help you.