The Road to Productionizing Enterprise Analytics
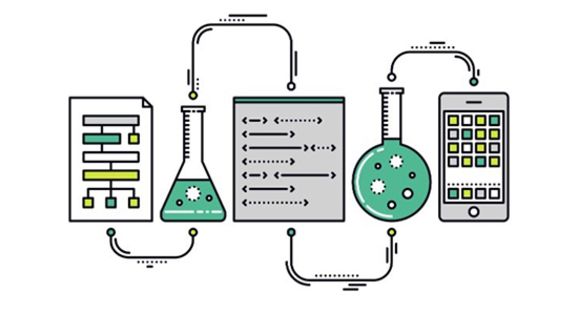
Companies are embracing investments in analytics and data science. Technology vendors are rapidly providing ever more capabilities. And yet, many enterprise organizations struggle to scale their analytics investments effectively to achieve the largest impact.
So why do organizations struggle with deploying and productionizing analytical insights and models?
Knowledge gaps by analysts
Analysts and data scientists typically have great statistical and modelling experience, but don’t necessarily have the software development background to progress their work to an enterprise level. Often they can come up with innovative solutions which work well in isolation, but which don’t scale to the degree required.
Many options of approach
More and more technology vendors are introducing analytical capabilities into their products. This not only raises questions around which vendors to use, but also where in the stack to deploy models: be that embedded in a self-service data exploration and visualization tool, in-database storage and processing, or any number of cloud offerings that can be called as a service.
Fast moving technologies and landscape
Technologies in this space are evolving very quickly, particularly the cloud-based ones. So it is difficult to keep up, and many worry that the choices they make now will be out-dated in one year, or three years’ time.
Combined, these factors can make the task of establishing enterprise-wide analytics solutions rather daunting. As a result, many organizations find themselves moving through three stages of analytics productionization. And as they progress, it becomes easier for analysts and data scientists to share their work with colleagues and maximise the impact.
Stage 1: Isolated Analysts
The first scenario is that of a lone data scientist working largely in isolation from IT. Or perhaps a team of data scientists each applying their own expertise to separate business problems. Because there is little oversight and everyone has their own way of working, there is a large amount of variability in methods and work may be unnecessarily slow.
Often people think they are doing “one-off” pieces of analysis, but once business stakeholders see value they want to replicate it for other geographies or product lines. There is then a real struggle to meet expectations and make a solution available as fast as desired. If you don’t establish the platform early, you’re most likely going to struggle.
Stage 2: Structured development environment
The next stage is a structured development environment. Analysts have appropriate tools provisioned by IT to handle the heavier compute requirements of analytical methods. They also have access to clean, accurate, official data sources.
In terms of both tools and practices, a structured environment will save time and ease the move to a production-ready solution.
Stage 3: Streamlined framework
The third step is a thought out, scalable and streamlined framework for developing and productionising analytical insights which allow the analyst to make the largest impact with their work, as well as being flexible to new technological advances in the future.
This would involve both automating the processing of data required for analysis, as well as scheduling and automating the algorithms and analyses themselves.
You would also want to focus on ensuing that productionization projects involve all three of business, IT and the analysts who have developed the solution, to make sure it is both technically realistic and thought through, as well as really meeting the needs of the business in the way they need.
So, how do you pull all this together successfully?
The most important thing is to put in place a framework that has been thought out and works for you. And though the development environment and productionized process are described here as being separate stages, they do not necessarily need to be implemented at different times or in different technologies, but it’s important to think of them as distinct logically and complementing each other in their functions.
Use the most appropriate tools and people for the job. By doing this you will enable analysts through appropriate technology and standards, and make moving from a pilot study to a full solution manageable.